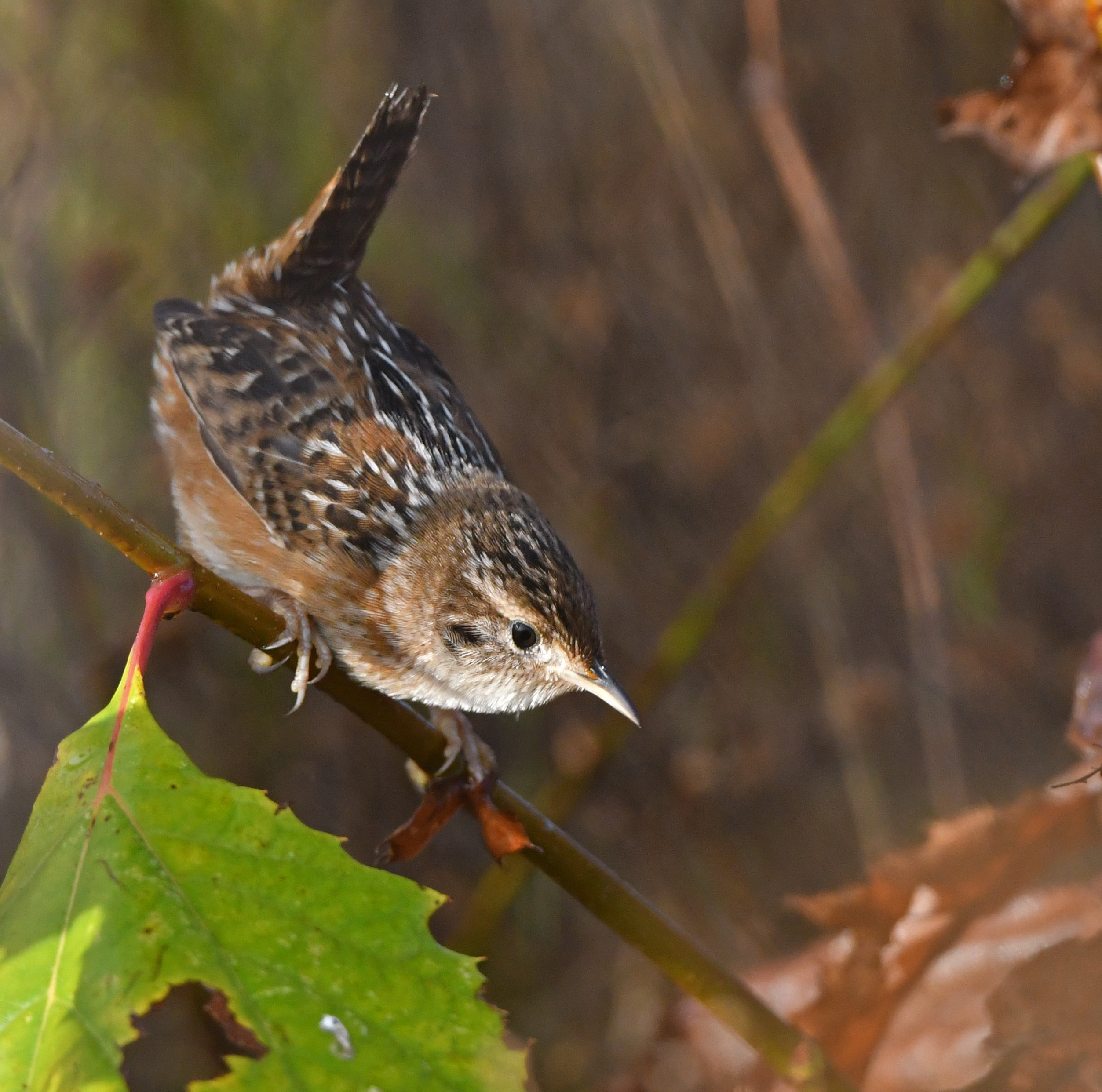
High-resolution models most accurately capture bird biodiversity patterns, new study finds
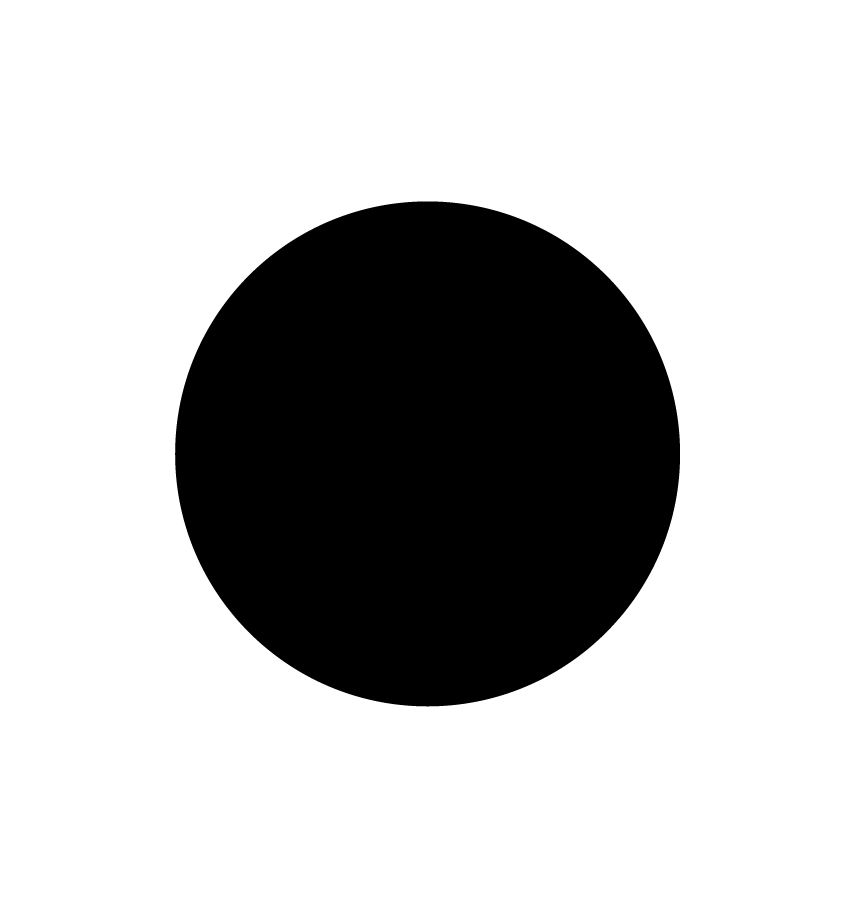
It may not be immediately obvious, but continental and global-scale maps of biodiversity patterns can be built from hundreds or thousands of individual estimates of species’ spatial distributions. Each of these distributions is modelled by connecting a set of occurrence points with multiple environmental variables, such as rainfall, temperature, and land cover type. With so much data feeding into one product, how can we be confident in the accuracy of large-scale biodiversity estimates?
Grain size, or modeling resolution, is an important factor in understanding the accuracy of these maps, according to Yale BGC Center researchers who recently published a paper titled “Fine-Grain Predictions Are Key to Accurately Represent Continental-Scale Biodiversity Patterns” in the journal Global Ecology and Biogeography. When scientists model species distributions, they have to decide if the environmental and species data will be spatially represented at a fine scale – like 1-kilometer grid cells – or a relatively coarse scale – like 10-kilometer grid cells.
“In the past, it has been more computationally feasible to fit these large-scale models at coarser resolution,” said Dr. Jeremy Cohen, Associate Research Scientist at Yale BGC and lead author of the study. “But with modern computing power and the increasing availability of high-resolution environmental data, we can now fit continental-scale models at very high resolution to make detailed predictions about relationships between species and habitat at local scales.”
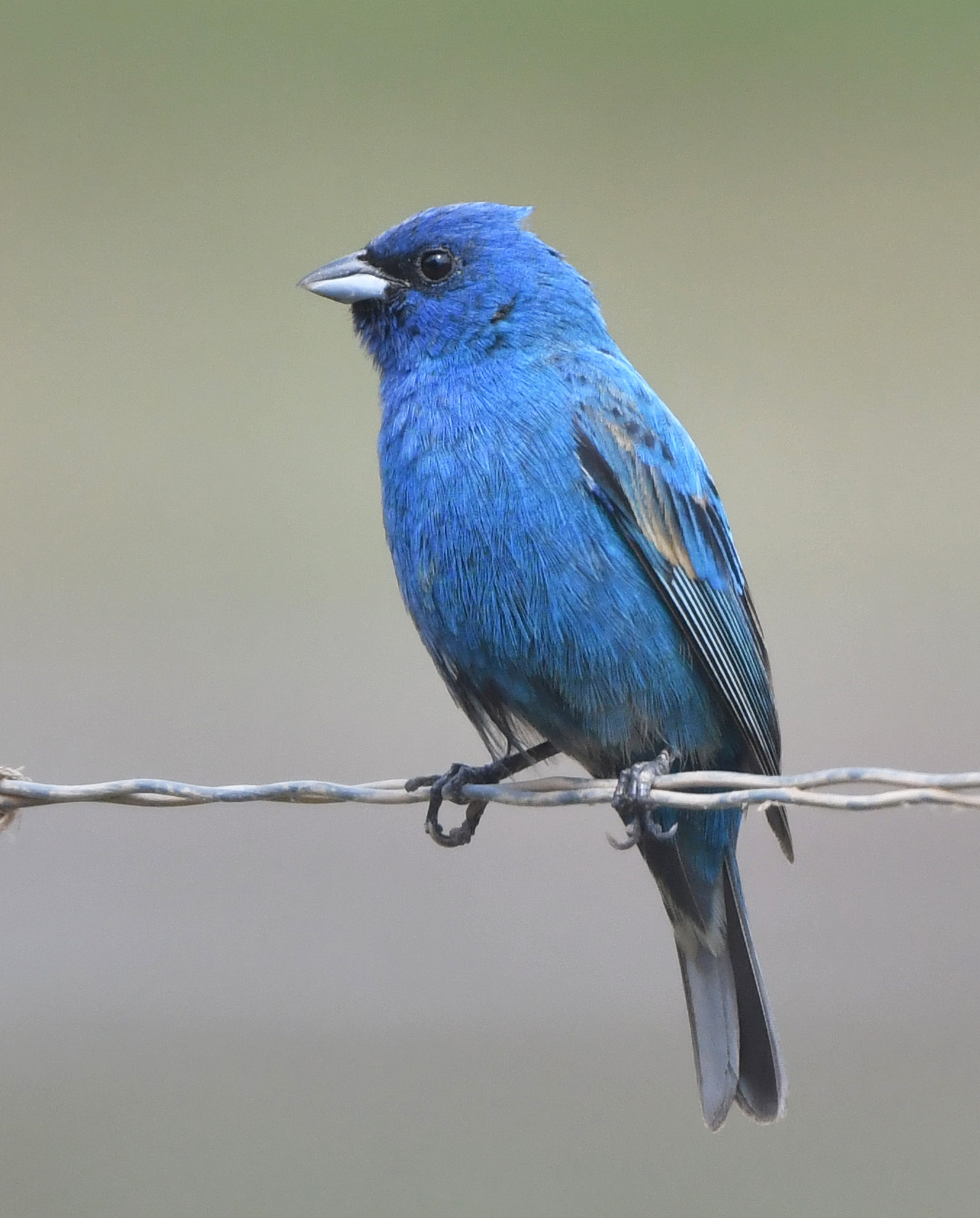
For a species that lives in a relatively homogenous ecosystem, such as the indigo bunting which occurs across the eastern deciduous forest, the choice of grain size might not make much of a difference in modeling outcomes; but, for a species that has specific habitat requirements across a patchier landscape, such as the lesser goldfinch which lives in the southwest deserts, coarsening the resolution risks mis-matching the occurrence points with the habitat variables. When aggregated to biodiversity maps, these species-level biases could lead to significant errors in predicted biodiversity distribution patterns that compound in desert or other regions.
While much research has focused on how the choice of grain size affects species distribution models (SDMs, models that match species observations with environmental information to predict distributions), few have examined how these grain-related biases differ across regions, species and seasons or how they impact large-scale biodiversity distribution maps.
In the new paper, researchers Cohen and Walter Jetz, a professor of Ecology and Evolutionary Biology at Yale and Director of the BGC Center, explore these grain size biases with North American birds. Using occurrence data from eBird, a citizen-science platform run by the Cornell Lab of Ornithology in which users from all over the world submit bird sightings, and a suite of environmental data layers, they modelled the summer and winter distributions of nearly 600 species across a range of grain sizes – from 1 to 50 kilometers – for a grand total of 4,955 individual SDMs.
“By leveraging tens of millions of high-resolution observations through eBird, we could model the distributions of nearly all US and Canadian bird species from high to low resolution and determine how the estimated range sizes and distributions in space change across different species and in different regions.” Cohen said. “We also wanted to explore seasonal differences in these biases by modeling across both summer and winter.”
With each species modeled, the researchers then aggregated the data to create biodiversity maps for both seasons at each grain size. Using holdover occurrence data from eBird that hadn’t been used in model development, they tested the accuracy of both the individual SDMs and the aggregated biodiversity maps across 322 independent test sites across North America. These sites were chosen from eBird hotspots with over 100 surveys during each season, which are locations that see heavy birder traffic and therefore have species lists which are more likely to be complete.
At the species level, Cohen and Jetz observed that on average, coarse grain models predicted less total range area for species compared to 1-kilometer models. This was especially true for species that live in heterogeneous landscapes in the prairie and desert ecosystems where small patches of suitable habitat were often lost at larger grain sizes.
“For example, the sedge wren, a prairie species breeding in the upper midwest and great plains, lost large amounts of its estimated breeding territory on the periphery of the range in coarse-resolution models where grassland habitat is much patchier than in the interior,” Cohen explained. “Therefore, coarse-resolution models might miss this important habitat and fail to lead to conservation action at the range edges, where species are often already living on the edge.”
By comparing the aggregated biodiversity maps across grain sizes, the researchers determined that increasing grain size tended to overpredict diversity metrics in the American west and in British Columbia and underpredict in the boreal forest, the prairie pothole region, and the American great plains, particularly in the summer – the winter models saw similar overprediction in the American west but not much underprediction. On the other hand, large, relatively continuous ecosystem types, notably the eastern deciduous forests, showed little change across grain size differences.
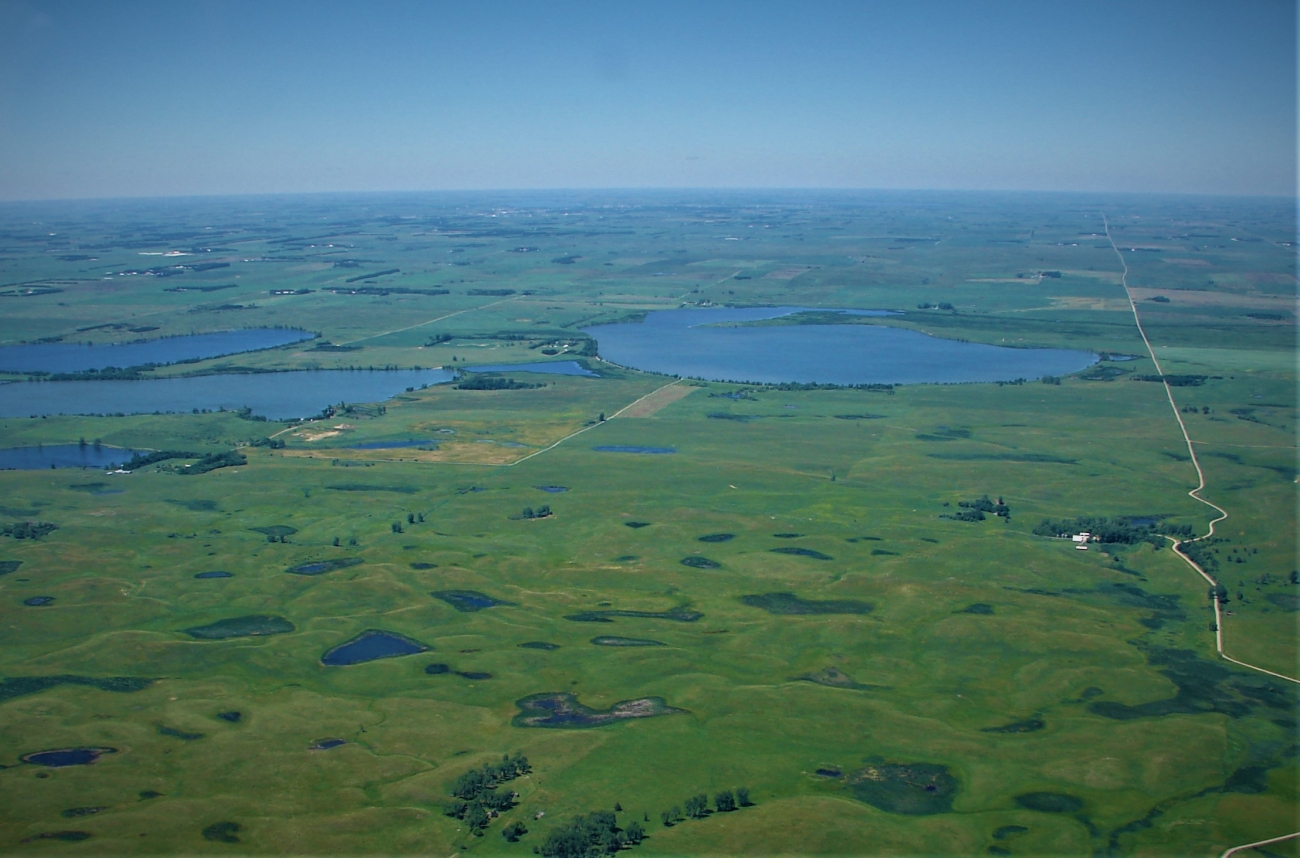
Furthermore, the 1-kilometer maps beat all other grain size models in terms of species accuracy using the 322 test sites. Deserts and scrublands in particular experienced the largest improvement in species accuracy as grain size decreased while grasslands and boreal and temperate forests were relatively less affected.
“Modeling at fine resolution may be more important in certain habitat types, including desert and grassland habitats, and these regions contain many isolated species with smaller ranges and very specific habitat requirements,” said Cohen.
Indeed, a crucial finding in this study is that grain size biases tend to mostly impact species of higher conservation concern: those who depend on patchy, non-continuous habitats or those with smaller, more restricted habitats. If conservation practitioners are making decisions about how to best manage land and protect important and vulnerable species, working off unreliable maps and data can severely hinder success and lead to resources not being allocated effectively.
“If we fail to make use of high-resolution data products and model species at fine grain, we may miss important habitat for sensitive species, leading to poorer conservation outcomes,” said Cohen.