Areas of Interest
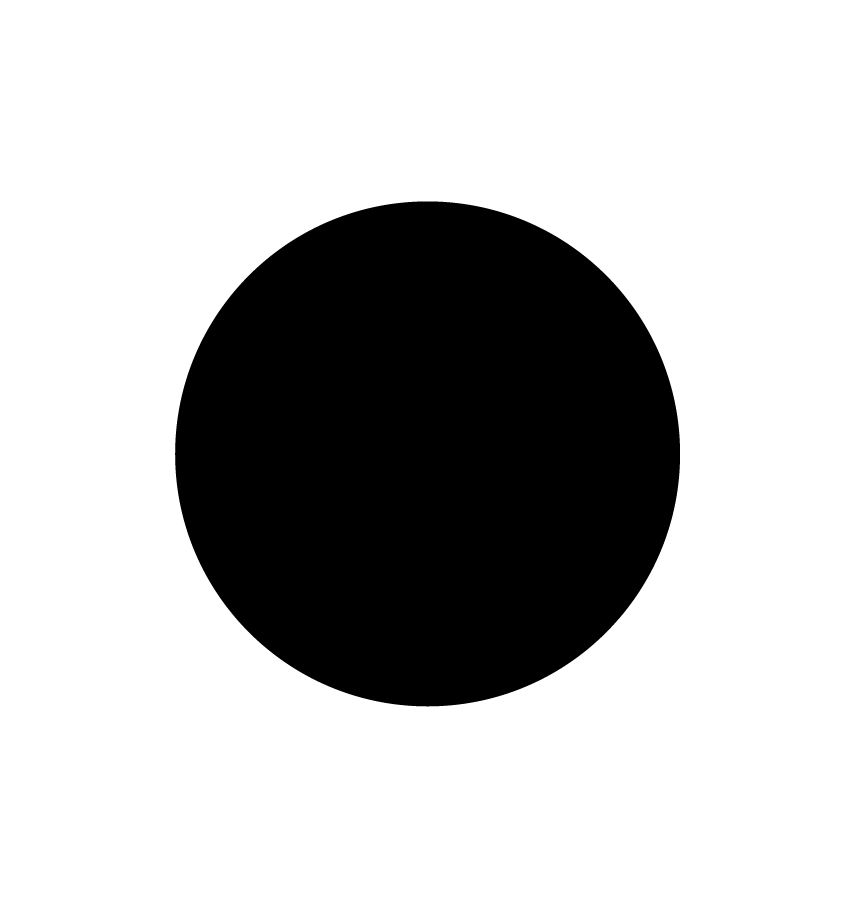
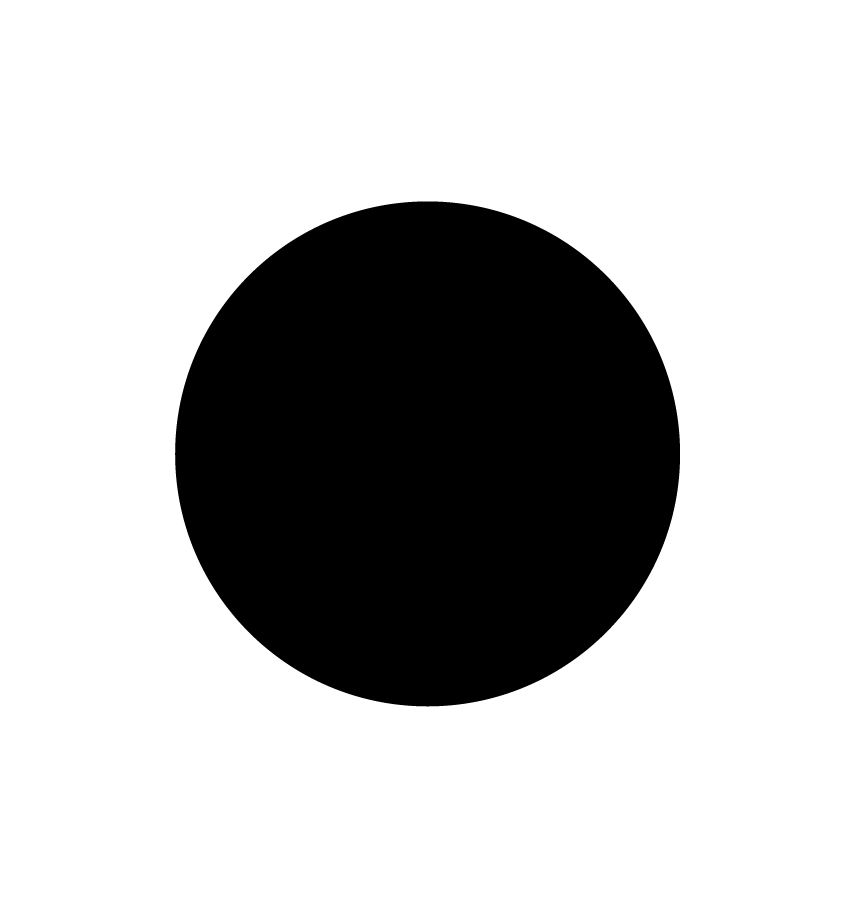
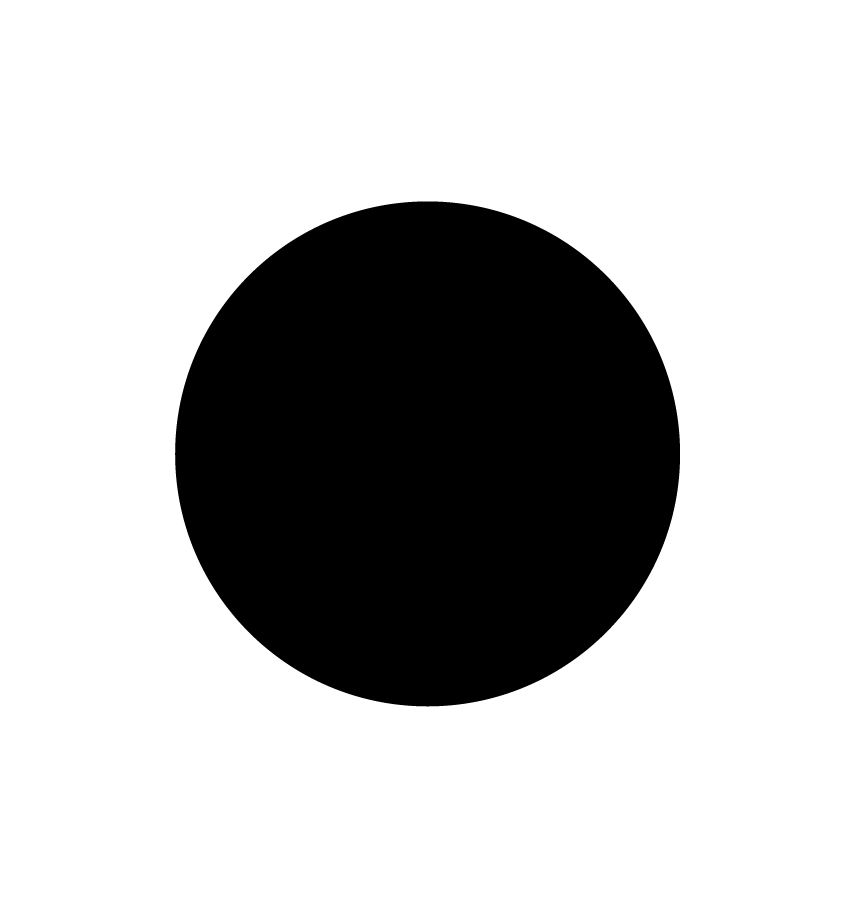
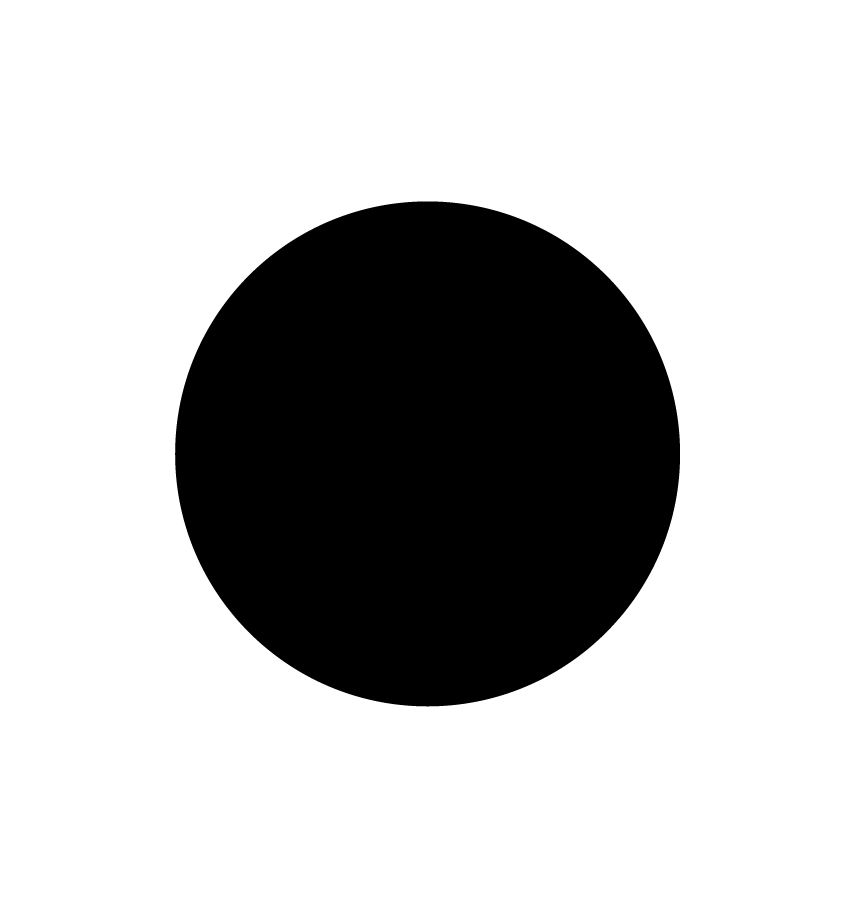
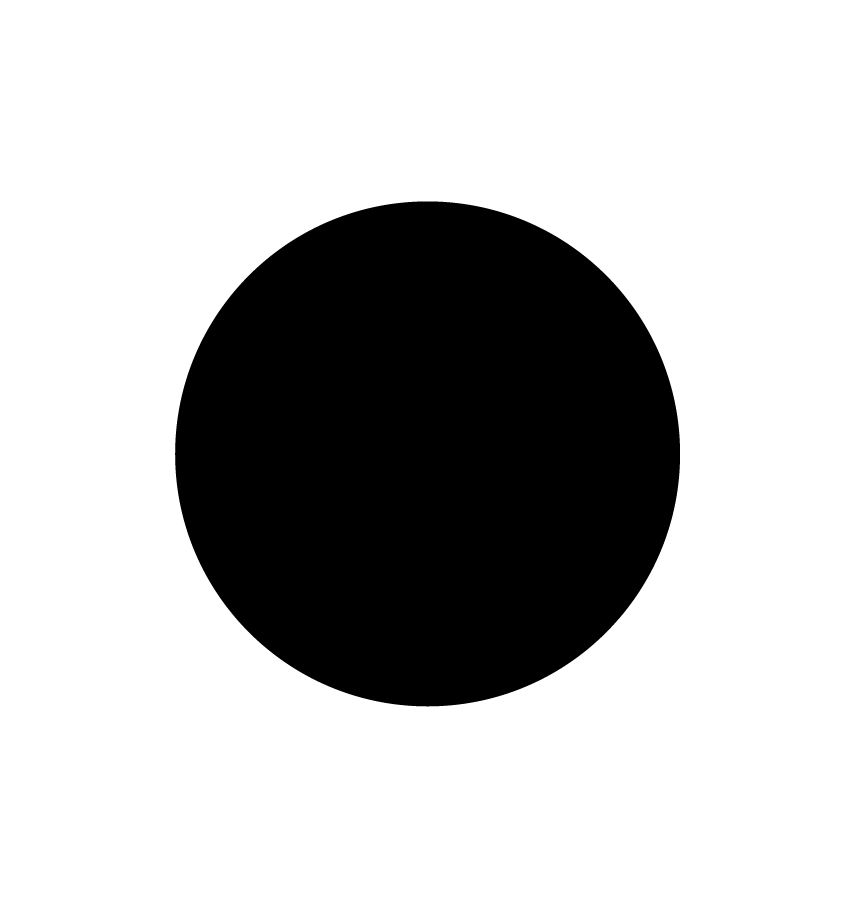
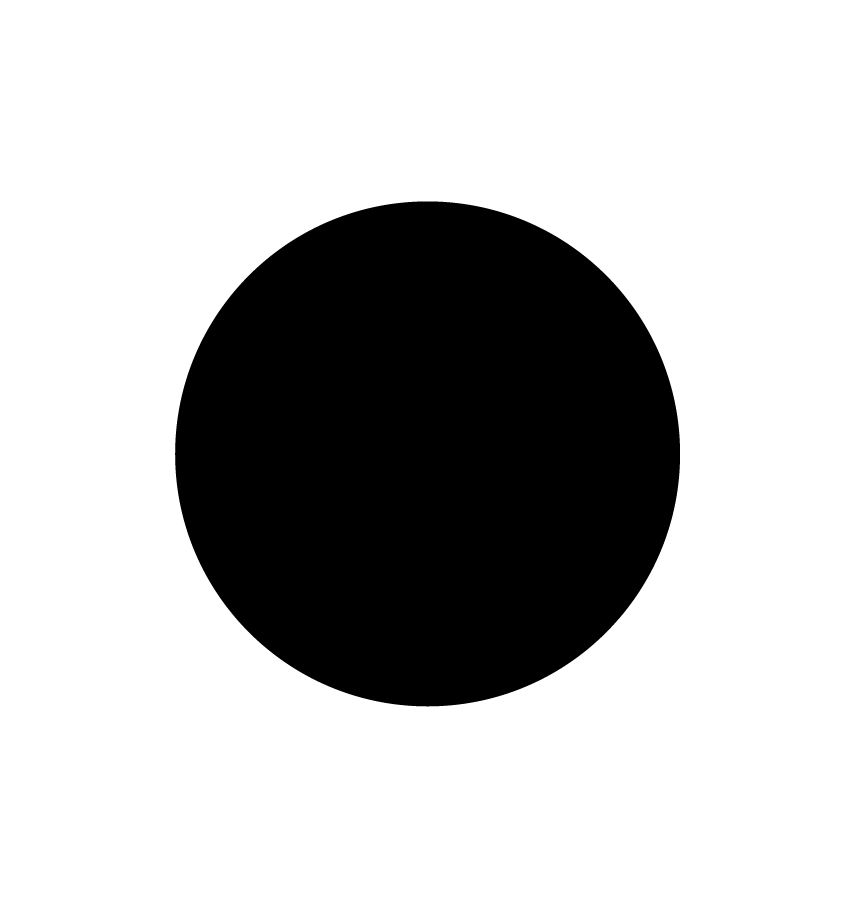
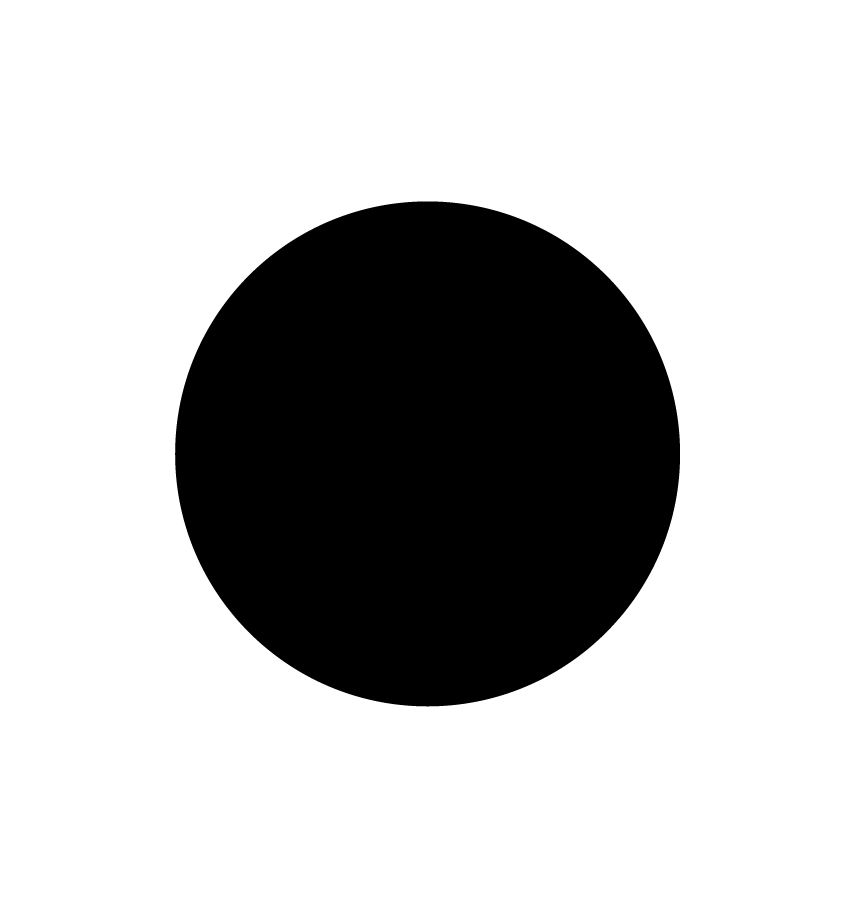
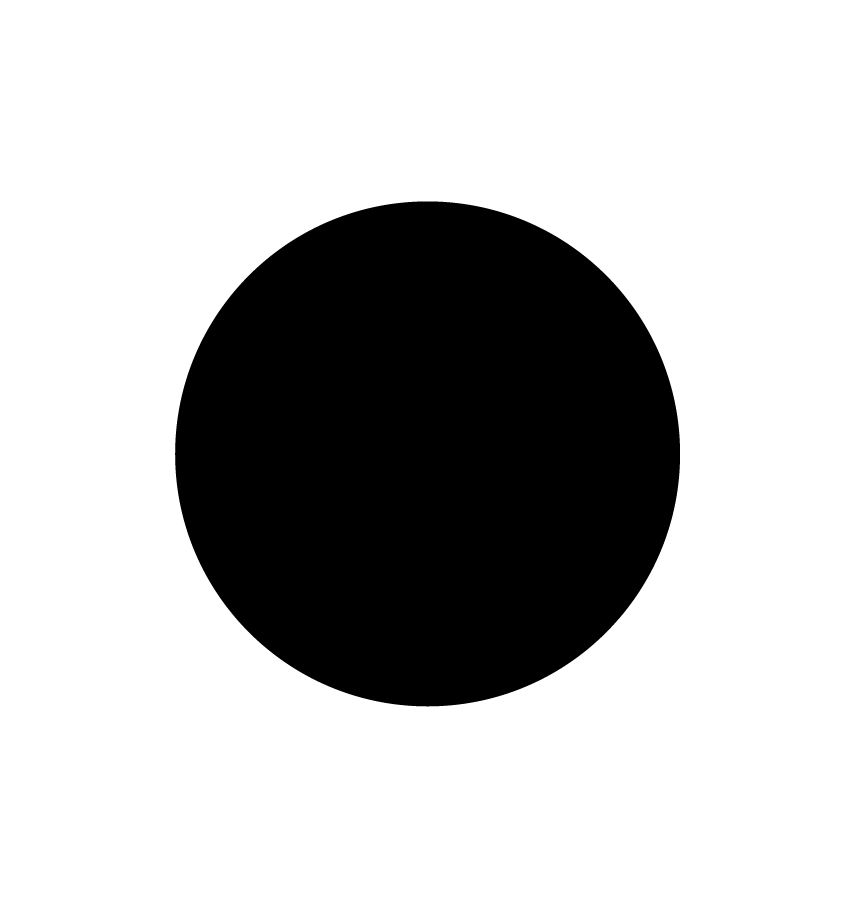
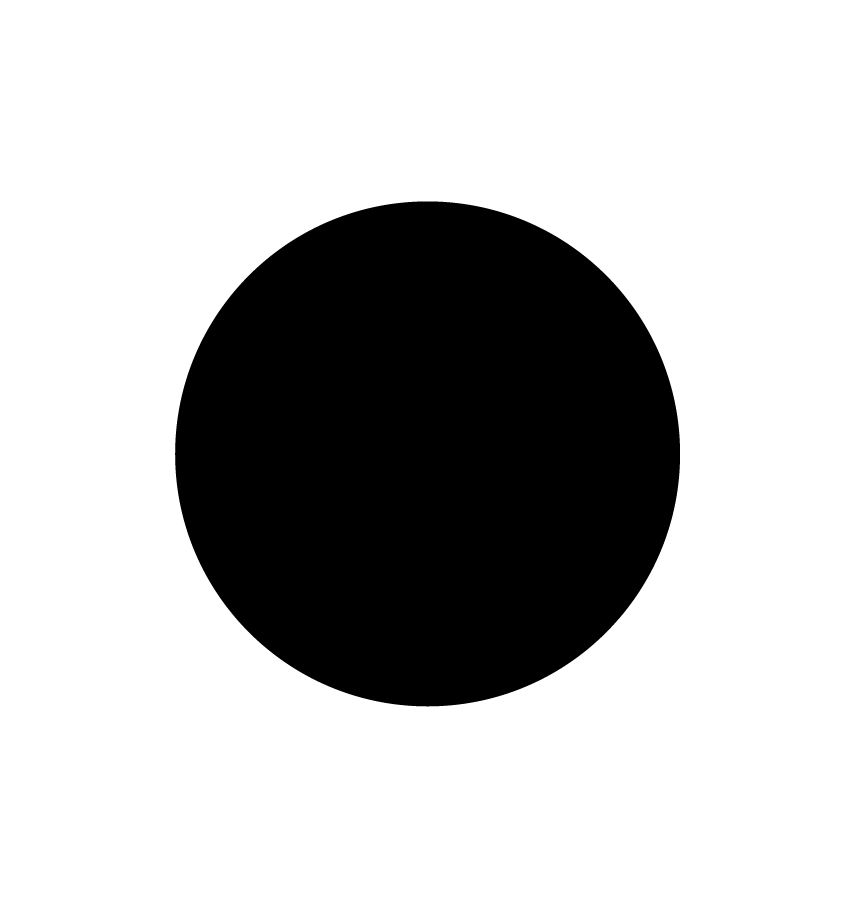
My research focuses on applying machine learning techniques to build more robust and mechanistic models of the global-scale patterns of biodiversity and species distributions. I am also interested in birth-death processes for population dynamics, niche modeling with animal movement data, and point-process models for metacommunity analysis. In general, I’m interested in developing models that unify ecological processes across individual and species/community scales, account for additional biases and confounding processes, and provide actionable insights for conservation practitioners and managers. Before coming to Yale, I completed my PhD in computer science at the University of Massachusetts, Amherst, where my research focused on novel demography models for populations of unmarked individuals and a corresponding suite of statistical inference methods for distributions with countably infinite support.
Woodworking, backpacking, scuba diving, video/board gaming, photography, gardening, pyrography
Beery S, E Cole, J Parker, P Perona, K Winner. 2021. Species Distribution Modeling for Machine Learning Practitioners: A Review. ACM SIGCAS Conference on Computing and Sustainable Societies. https://doi.org/10.1145/3460112.3471966
Winner K, MJ Noonan, CH Fleming, KA Olson, T Mueller, D Sheldon, JM Calabrese. 2018. Statistical inference for home range overlap. Methods in Ecology and Evolutionary Biology. https://doi.org/10.1111/2041-210X.13027 (pdf)
Winner K, D Sujono, D Sheldon. 2017. Exact Inference for Integer Latent-Variable Models. Proceedings of Machine Learning Research. Available from https://proceedings.mlr.press/v70/winner17a.html.
Winner K, D Sheldon. 2016. Probabilistic Inference with Generating Functions forPoisson Latent Variable Models. Advances in Neural Information Processing Systems. (pdf).


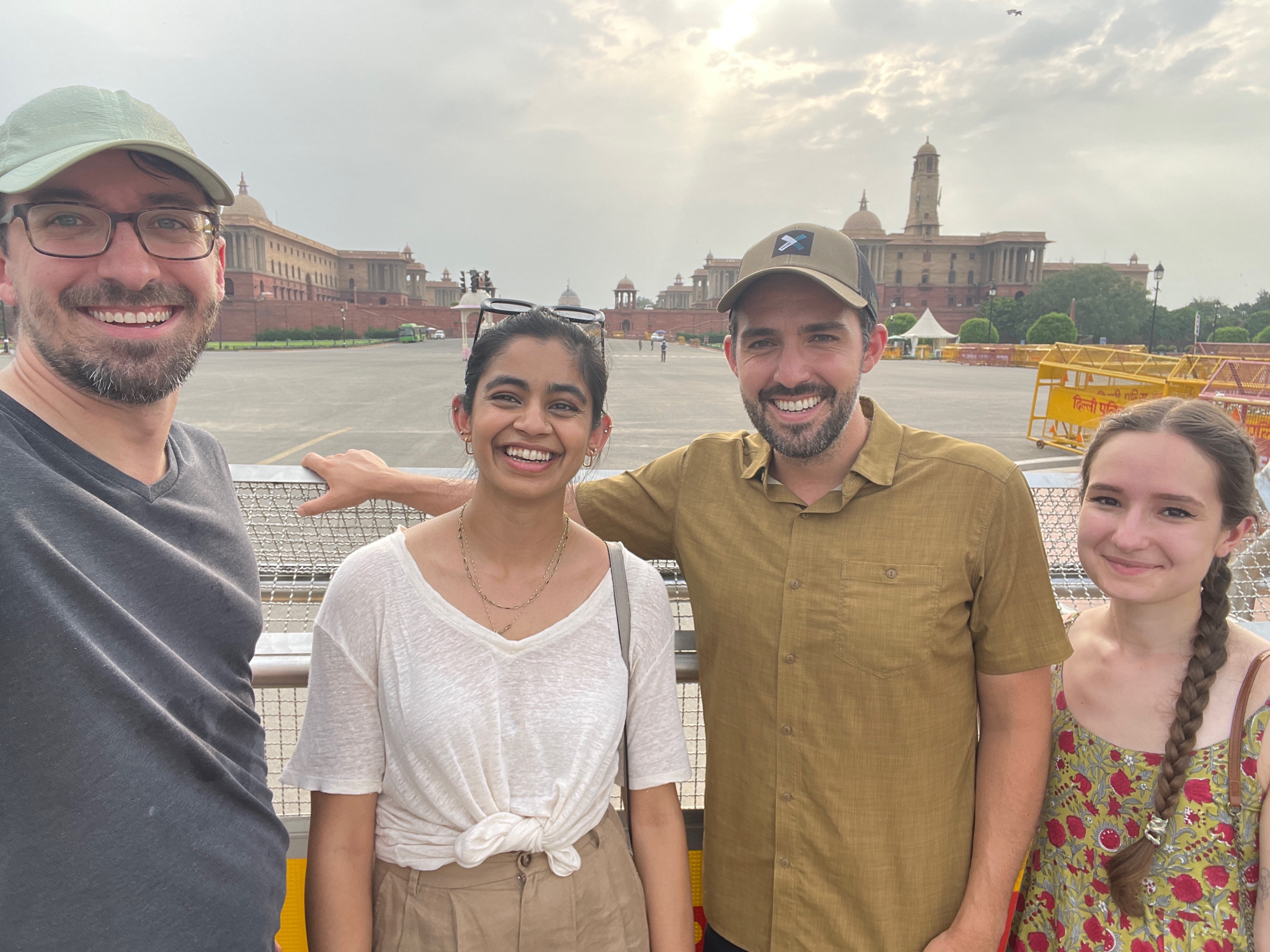